Salmonella is a complex pathogen that is represented by over 2,600 different serovars. These serovars are classified based on their unique combination of somatic (O) and flagellar (H) antigens. Underlying this antigenic diversity is remarkable genetic diversity. The genetic plasticity of individual serovars gives rise to a host of unique phenotypes such as serovars that are host restricted and host adapted. For example, serovar Typhi, the causative agent of typhoid, is restricted to humans, while serovar Gallinarum is restricted to poultry, where it causes fowl typhoid. Serovar Dublin is an example of a host-adapted serovar; while it is commonly associated with bovine hosts, it can occasionally cause illness in humans. Conversely, serovar Typhimurium is an example of an unrestricted serovar that can colonize many different hosts. Similarly, some serovars have a greater association with human illness than other serovars: Enteritidis and Typhimurium are collectively responsible for nearly a quarter of salmonellosis cases each year in the U.S.,1 while serovar Kentucky is rarely linked to human illness.2 Highly pathogenic serovars possess an array of virulence factors that allow them to evade host immune responses and to induce pathogenesis.
Each year in the U.S., there are over 1.35 million cases of salmonellosis, 23,000 hospitalizations, and 450 deaths, along with an estimated annual economic burden of $3.7 billion.3,4 According to the Interagency Food Safety Analytics Collaboration (IFSAC), most (> 75 percent) Salmonella outbreaks can be attributed to seven specific food categories, including:
- Chicken (18.6 percent)
- Fruits (15.8 percent)
- Pork (12.1 percent)
- Seeded vegetables (10.9 percent)
- Other produce (9.4 percent)
- Beef (6.5 percent)
- Turkey (5.5 percent).5
This source diversity highlights the complexity of understanding Salmonella outbreaks, as other bacterial foodborne pathogens are attributed to fewer sources. The estimated attribution of salmonellosis cases to chicken has increased from 12.7 percent in 2016 to 18.6 percent in 2021 (Figure 1). As part of its Salmonella verification program, the U.S. Department of Agriculture's Food Safety and Inspection Service (USDA-FSIS) monitors Salmonella in poultry processing establishments. Publicly available data from this program has demonstrated that the poultry industry has reduced Salmonella prevalence by over 50 percent in chicken parts from 16.7 percent to 7.6 percent over the same time frame (Figure 1).6 Thus, actions by the poultry industry that have effectively reduced Salmonella incidence have not translated into reduced human illnesses that are linked to the consumption of contaminated poultry.

According to USDA-FSIS data, the most common serovars found in broiler carcasses and parts at processing between 2016 and 2023 are Kentucky (55.1 percent in carcasses, 33.4 percent in parts), Enteritidis (13.9 percent in carcasses, 26.7 percent in parts), and Typhimurium (10.8 percent in carcasses, 11.6 percent in parts).6 Serovar Kentucky is also the most common serovar isolated from breeder flocks.7 To effectively control Salmonella in chicken, there is a need to understand where specific serovars of concern originate and how they are adapted to persist within broiler production and processing.
Broiler production in the U.S. is vertically integrated, with each stage of production from breeding and hatching to processing and distribution maintained within a single complex that belongs to a single company. This approach allows for greater control and coordination across the entire supply chain, leading to more efficient production and distribution and also to improved food safety and quality control. With respect to Salmonella transmission, strains that are found in breeders have also been found at processing, demonstrating that Salmonella can move throughout a complex.8
Food safety experts excel in precise characterization of Salmonella isolates, and this is often accomplished by whole genome sequencing (WGS) or other molecular approaches. However, the isolation of these strains in the first place is limited for two important reasons:
- Conventional culture-based methods rely on selection of a small number of colonies (typically 1–3 colonies) from Salmonella indicator plates (e.g., XLT-4) that are characterized and reported. Statistically, these colonies represent the most abundant serovar, and thus, in mixed Salmonella populations, surveillance is biased toward the most abundant serovar. Importantly, lower-abundance serovars remain masked and unreported. This can be mitigated in part where careful attention is paid to selecting colonies with different morphologies.
- Salmonella detection relies on a two-step culturing process of non-selective pre-enrichment followed by selective enrichment. Pre-enrichment enables resuscitation and growth of damaged Salmonella before they are transferred into a selective enrichment broth, typically performed in buffered peptone water (BPW). Selective enrichment is usually performed in Rappaport-Vasiliadis (RV) and tetrathionate (TT) broths, where Salmonella can proliferate while the growth of other microbes is suppressed. Different serovars do not grow equally in these different enrichment broths, and this can introduce sample detection bias.9 The implications of this are significant: where multiple serovars are present on a broiler carcass, for instance, the serovar that is ultimately detected following culture isolation may not reflect the most prevalent serovar present on the carcass itself (Figure 2).

Salmonella surveillance is biased toward serovars that are abundant in the original sample, and/or those that grow better under selective enrichment (Figure 3). When a serovar of concern is undetected, the opportunities to understand where Salmonella is originating from or where and how it is persisting on a farm or at processing are lost, ultimately limiting the efficacy of Salmonella controls.
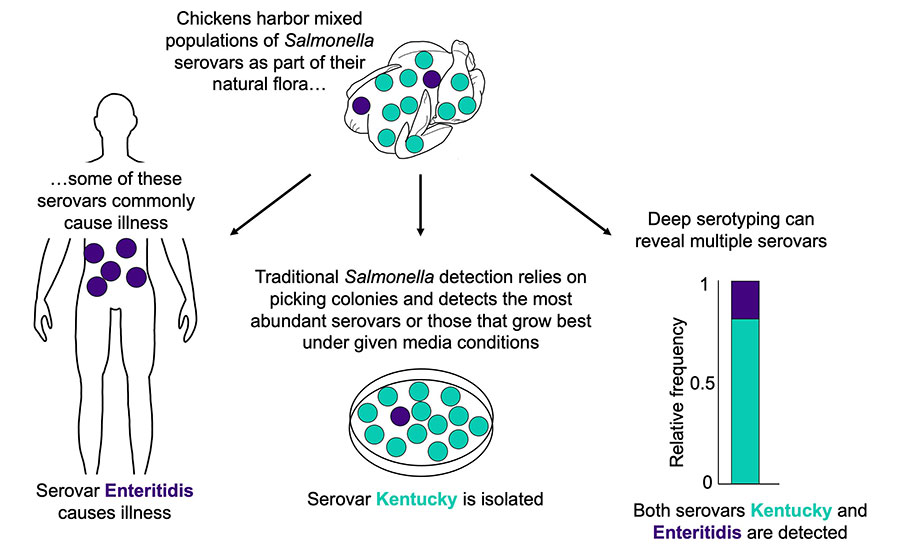
To overcome these challenges, there is a need for methodology that can resolve mixed serovar populations. The first deep serotyping method developed was CRISPR-SeroSeq (serotyping by sequencing the clustered regularly interspaced palindromic repeats), which can identify multiple serovars present in a mixed Salmonella population10 (Figure 3). CRISPR-SeroSeq is an amplicon-based, next-generation sequencing methodology that uses the sequences of the native CRISPR arrays in Salmonella for serotyping. Since Salmonella CRISPR spacer sequences correlate with serovar identity,11,12,13 the resulting sequence information can be used to identify each serovar. Furthermore, by using sequence read frequency, the relative abundance of each serovar in a mixed serovar sample can also be determined.
Deep Serotyping Shows that Poultry Interventions Reduce Salmonella Complexity
CRISPR-SeroSeq has been utilized in different studies investigating Salmonella populations in broiler production and processing. On the pre-harvest side, one study assayed 134 environmental samples from commercial breeder houses across Georgia and found that 32.1 percent of Salmonella-positive samples contained multiple serovars, with an average of 1.6 serovars per sample.7 A subsequent study performed in four broiler complexes determined that of 67 Salmonella-positive environmental samples from broiler houses, 57 percent contained multiple serovars (with an average of 1.7 per sample).14 This intuitively makes sense: given their value and longevity, biosecurity is tighter on breeder flocks, and this limits Salmonella transmission. Furthermore, for most broiler producers in the U.S., vaccination against Salmonella is performed exclusively in breeder flocks, not broilers.
On the processing side, a study examined serovar complexity between rehang carcasses (n = 153) and post-chill carcasses (n = 38) and found significantly higher complexity in rehang carcasses.15 Here, 48.4 percent of rehang carcasses contained two or more serovars, compared to 7.9 percent of post-chill carcasses. Again, this also makes sense: chilling is the most effective antimicrobial step in processing; therefore, it follows that a reduction in Salmonella prevalence (65.8 percent versus 4.7 percent for rehang and post-chill, respectively) is accompanied by a reduction in serovar complexity, as well. Collectively, these studies show that serovar complexity differs along the poultry production chain and, importantly, that interventions that reduce Salmonella prevalence also reduce serovar complexity.
Deep Serotyping Highlights Media Bias for Salmonella Isolation
Salmonella isolation requires selective enrichment. RV broth provides a selective environment due to its low pH and high osmolarity, plus the addition of malachite green, which collectively inhibit background microflora. TT is an electron acceptor for anaerobic respiration in Salmonella due to the presence of tetrathionate reductase, enabling Salmonella to compete against non-Salmonella in TT broth. TT broth contains bile salts, which cause DNA damage, and some formulations contain brilliant green, which inhibits E. coli, Proteus spp., and Gram-positive bacteria.
Given that Salmonella serovars can have different phenotypes, it is not surprising that this can manifest in their ability to grow differently in different selective broths.9 These differences are only apparent when Salmonella is growing in mixed-serovar populations where the competition between serovars can be observed. More recently, the application of CRISPR-SeroSeq to identify serovar profiles in samples enriched in parallel in RV and TT broths has also highlighted differences. Here, deep serotyping performed on broiler carcasses at processing identified 18 carcasses containing multiple serovars, including serovar Enteritidis. Importantly, in 11 of these, serovar Enteritidis was detected following enrichment in TT broth, but not in RV broth, and in six carcasses, the relative abundance of Enteritidis was lower in RV than in TT.16
Similarly, in that same study, there were 34 carcasses containing multiple serovars, including serovar Infantis. In 28 of these carcasses, the relative proportion of Infantis was lower in TT than in RV. Furthermore, differences in serovar recovery were also observed when different plating media were used. Therefore, choice of selective media can skew which serovars are detected or recovered. Where deep serotyping is used, the probability of detecting all serovars is obviously higher than by picking individual colonies. The resolution of deep serotyping depends on the depth of sequencing and the extent to which any media bias is occurring. The latter could be driven by the combination of different serovars or, potentially, by other microbes that are also present.
Applications for Deep Serotyping to Improve Food Safety in Poultry Production
Looking forward, there are several applications where a deep serotyping approach can provide a useful tool for improving food safety in poultry production.
Autogenous vaccine development to control specific serovars. Currently in the U.S., commercially available Salmonella vaccines target serovars Typhimurium (attenuated live vaccines) and Enteritidis (killed vaccine). In addition, autogenous vaccines are killed vaccines that are generated against specific strains found in a particular company or complex and are generally restricted for use within that same complex. This provides greater flexibility for producers to target additional serovars. Autogenous vaccines can be delivered using a mixture that contains mixed isolates to target multiple serovars.
Selection of a Salmonella strain to generate an autogenous vaccine prioritizes serovars that are found in the processing plant, as these are candidates for serovars that are escaping current interventions. Individual isolates are then characterized to identify those that may confer the greatest food safety risk (for example, multidrug-resistant strains or strains with a greater suite of virulence factors), and these are used to generate the vaccine itself. However, there is a need to confirm whether that serovar (or strain) is also present in live production facilities, as well. In the absence of this information, there is not a strong rationale for administering a vaccine to birds. Serovar Kentucky is well adapted to grow in chickens,17 and a study in breeders showed that in 80 percent of Salmonella-positive flocks, the colony isolated was serotyped as Kentucky.7 Therefore, it is likely that serovar Kentucky is outcompeting other serovars. Deep serotyping provides a high-resolution approach for screening live production samples to confirm the presence of serovars of concern so that they can be included in autogenous vaccines. When applied to breeders, this method determined that when serovar Infantis was present, 90 percent of the time, it was outnumbered by another serovar, although not always by Kentucky.7
Biomapping to understand Salmonella persistence and transmission. In the context of food safety, biomapping includes tracking the presence of Salmonella or other foodborne pathogens. At post-harvest, biomapping can be useful to monitor antimicrobial interventions and evaluate process control.18,19 At pre-harvest, biomapping for specific serovars is useful for determining where and how these serovars are entering a broiler complex (e.g., feed, rodents, breeders) and where they are persisting (e.g., litter, rodents, beetles). This information can be used to implement Salmonella controls in a targeted manner. In combination with subtyping tools that can provide information regarding specific strains, biomapping can also be used to ascertain whether specific strains are being transmitted through a complex. As described above, where traditional Salmonella isolation methods are used, biomapping will be limited to only identifying the most prevalent serovar(s). Deep serotyping can be used broadly on a subset of samples to identify serovars of concern in a complex. This could then be followed with targeted approaches looking for specific serovars such as through the use of serovar-specific qPCR assays,20 although the availability of such tools is currently limited.
Predictive forecasting to enable proactive Salmonella control. The prevalence of specific and dominant serovars in poultry has shifted, and many of these shifts occurred in response to mitigation of a specific serovar.21 Elimination of serovar Gallinarum (and its biovar, Pullorum) due to fowl typhoid decades ago created a vacant niche that was subsequently filled by the genetically similar serovar Enteritidis. Efforts to mitigate serovar Enteritidis enabled serovar Kentucky to increase in frequency, and the latter has been the top serovar in poultry for a number of years. The reduction of serovars Typhimurium and Heidelberg in poultry over the last decade is generally attributed to the widespread use of live-attenuated serovar Typhimurium vaccines.
In 2018, an outbreak occurred that was caused by serovar Infantis. Importantly, the outbreak strain was identified across multiple different processors, suggesting that this serovar was present broadly across the industry. Prior to the outbreak, this serovar was infrequently detected by USDA-FSIS; in 2017, only 13 percent of Salmonella positive parts were typed as serovar Infantis. Since that time, Infantis has increased in prevalence and, in 2021, was the most frequent serovar detected from parts, with over one third of Salmonella-positive parts being attributed to serovar Infantis. This monitoring relies on picking and characterizing a single isolate; therefore, a serovar must be consistently present at a high relative frequency to be reliably identified.
Due to its high-resolution capacity, a monitoring approach that utilizes deep serotyping would afford the possibility to detect serovars of concern earlier than they may be repeatedly detected by culture-based approaches. Early identification would provide industry time to proactively target this serovar before it becomes a significant problem. This could include developing serovar-specific controls (e.g., vaccinations) or confirming whether it is susceptible to antimicrobial interventions used in the plant.
Summary
Regarding poultry production and processing, deep serotyping is a high-resolution technology that can be applied in different scenarios at pre- and post-harvest to improve food safety. Furthermore, the ideas presented here can translate to other food animal production systems.
References
- Centers for Disease Control and Prevention (CDC). "National Outbreak Reporting System (NORS)." Accessed April 22, 2024. https://wwwn.cdc.gov/norsdashboard/.
- Richards, A.K., S. Kue, C.G. Norris, and N.W. Shariat. "Genomic and Phenotypic Characterization of Salmonella enterica Serovar Kentucky." Microbial Genomics 9, no. 9 (2023). https://pubmed.ncbi.nlm.nih.gov/37750759/.
- Tack, D.M., E.P. Marder, P.M. Griffin, et al. "Preliminary Incidence and Trends of Infections with Pathogens Transmitted Commonly Through Food—Foodborne Diseases Active Surveillance Network, 10 U.S. Sites, 2016–2019." CDC. Morbidity and Mortality Weekly Report 69 (2020). https://onlinelibrary.wiley.com/doi/full/10.1111/ajt.15412.
- Hoffmann, S., M.B. Batz, and J.G. Morris. "Annual Cost of Illness and Quality-Adjusted Life Year Losses in the United States due to 14 Foodborne Pathogens." Journal of Food Protection 75, no. 7 (2012): 1292–1302. https://pubmed.ncbi.nlm.nih.gov/22980013/.
- U.S. Department of Health and Human Services (DHHS), CDC, U.S. Food and Drug Administration (FDA), and U.S. Department of Agriculture Food Safety and Inspection Service (USDA-FSIS). "Foodborne Illness Source Attribution Estimates for Salmonella, Escherichia coli O157, Listeria monocytogenes, and Campylobacter using Outbreak Surveillance Data, United States." Interagency Food Safety Analytics Collaboration (IFSAC), 2022.
- USDA-FSIS. "Raw Poultry Sampling." April 5, 2024. https://www.fsis.usda.gov/news-events/publications/raw-poultry-sampling.
- Siceloff, A.T., D. Waltman, and N.W. Shariat. "Regional Salmonella Differences in United States Broiler Production from 2016 to 2020 and the Contribution of Multiserovar Populations to Salmonella Surveillance." Applied and Environmental Microbiology 88, no. 8 (2022). https://pubmed.ncbi.nlm.nih.gov/35384708/.
- Liljebjelke, K.A., C.L. Hofacre, T. Liu, D.G. White, S. Ayers, S. Young, and J.J. Maurer. "Vertical and Horizontal Transmission of Salmonella Within Integrated Broiler Production System." Foodborne Pathogens and Disease 2, no. 1 (2005): 90–102. https://pubmed.ncbi.nlm.nih.gov/15992303/.
- Gorski, L. "Selective Enrichment Media Bias the Types of Salmonella enterica Strains Isolated from Mixed Strain Cultures and Complex Enrichment Broths." PLoS One 7, no. 4 (2012): e34722.
- Thompson, C.P., A.N. Doak, N. Amirani, E.A. Schroeder, J. Wright, S. Kariyawasam, R. Lamendella, and N.W. Shariat. "High-resolution Identification of Multiple Salmonella Serovars in a Single Sample by Using CRISPR-SeroSeq." Applied and Environmental Microbiology 84, no. 21 (2018): 1–13. https://pubmed.ncbi.nlm.nih.gov/30170999/.
- Liu, F., R. Barrangou, P. Gerner-Smidt, E.M. Ribot, S.J. Knabel, and E.G. Dudley. "Novel Virulence Gene and Clustered Regularly Interspaced Short Palindromic Repeat (CRISPR) Multilocus Sequence Typing Scheme for Subtyping of the Major Serovars of Salmonella enterica subsp. enterica." Applied and Environmental Microbiology 77, no. 6 (2011): 1946–1956. https://pubmed.ncbi.nlm.nih.gov/21278266/.
- Fabre, L., J. Zhang, G. Guigon, S. Le Hello, V. Guibert, M. Accou-Demartin, et al. "CRISPR Typing and Subtyping for Improved Laboratory Surveillance of Salmonella Infections." PLoS One 7, no. 5 (2012): e36995. doi:10.1371/journal.pone.0036995.
- Shariat, N., R.E. Timme, J.B. Pettengill, R. Barrangou, and E.G. Dudley. "Characterization and Evolution of Salmonella CRISPR-Cas Systems." Microbiology 161, no. 2 (2015): 374–386. https://pubmed.ncbi.nlm.nih.gov/25479838/.
- Obe, T., A.T. Siceloff, M.G. Crowe, H.M. Scott, and N.W. Shariat. "Combined Quantification and Deep Serotyping for Salmonella Risk Profiling in Broiler Flocks." Applied and Environmental Microbiology 26, no. 89 (2023). https://pubmed.ncbi.nlm.nih.gov/36920215/.
- Richards, A.K., A.T. Siceloff, M. Simmons, G.E. Tillman, and N.W. Shariat. "Poultry Processing Interventions Reduce Salmonella Serovar Complexity on Postchill Young Chicken Carcasses as Determined by Deep Serotyping." Journal of Food Protection 87, no. 3 (2024): 100208. https://pubmed.ncbi.nlm.nih.gov/38142825/.
- Gorski, L., N.W. Shariat, A.K. Richards, A.T. Siceloff, A. Aviles Noriega, and D.M. Harhay. "Growth Assessment of Salmonella enterica Multi-serovar Populations in Poultry Rinsates with Commonly Used Enrichment and Plating Media." Food Microbiology 119 (May 2024). https://pubmed.ncbi.nlm.nih.gov/38225041/.
- Cheng, Y., A.A. Pedroso, S. Porwollik, M. McClelland, M.D. Lee, T. Kwan, K. Zamperini, V. Soni, H.S. Sellers, S.M. Russell, and J.J. Maurer. "rpoS-Regulated Core Genes Involved in the Competitive Fitness of Salmonella enterica Serovar Kentucky in the Intestines of Chickens." Applied and Environmental Microbiology 81, no. 2 (January 2015): 502–514. https://pubmed.ncbi.nlm.nih.gov/25362062/.
- Boubendir, S., J. Arsenault, S. Quessy, A. Thibodeau, P. Fravalo, W.P. Thériault, S. Fournaise, and M.-L. Gaucher. "Salmonella Contamination of Broiler Chicken Carcasses at Critical Steps of the Slaughter Process and in the Environment of Two Slaughter Plants: Prevalence, Genetic Profiles, and Association with the Final Carcass Status." Journal of Food Protection 84, no. 2 (2020): 321–332. https://pubmed.ncbi.nlm.nih.gov/33513257/.
- De Villena, J.F., D.A. Vargas, R.B. López, D.R. Chávez-Velado, D.E. Casas, R.L. Jiménez, and M.X. Sanchez-Plata. "Bio-Mapping Indicators and Pathogen Loads in a Commercial Broiler Processing Facility Operating with High and Low Antimicrobial Intervention Levels." Foods 11, no. 6 (2022). https://pubmed.ncbi.nlm.nih.gov/35327198/.
- Richards, A.K., B.A. Hopkins, and N.W. Shariat. "Conserved CRISPR Arrays in Salmonella enterica Serovar Infantis can Serve as qPCR Targets to Detect Infantis in Mixed Serovar Populations." Letters in Applied Microbiology 71, no. 2 (2020): 138–145. https://pubmed.ncbi.nlm.nih.gov/32333808/.
- Foley, S., R. Nayak, I. Hanning, T. Johnson, J. Han, and S. Ricke. "Population Dynamics of Salmonella enterica Serotypes in Commercial Egg and Poultry Production." Applied and Environmental Microbiology 77, no. 13 (2011): 4273–4279. https://pubmed.ncbi.nlm.nih.gov/21571882/.
- Interagency Food Safety Analytics Collaboration. "Annual Reports on Foodborne Illness Source Attribution Estimates." Last reviewed November 6, 2023. https://www.cdc.gov/ifsac/php/annual-reports/index.html.
Nikki Shariat, Ph.D. is Assistant Professor at the Poultry Diagnostic and Research Center in the Department of Population Health at the College of Veterinary Medicine at the University of Georgia. She holds a Ph.D. in Biological Sciences from Vanderbilt University and a B.Sc. degree in Molecular Biology and Genetics from the University of East Anglia in the UK. She is author or co-author on more than 40 published scientific papers, as well as the author of a book chapter in Salmonella: Methods and Protocols (Methods in Molecular Biology).